Red de Bibliotecas Virtuales de Ciencias Sociales en
América Latina y el Caribe
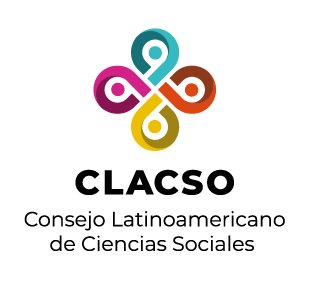
Por favor, use este identificador para citar o enlazar este ítem:
https://biblioteca-repositorio.clacso.edu.ar/handle/CLACSO/128040
Registro completo de metadatos
Campo DC | Valor | Lengua/Idioma |
---|---|---|
dc.creator | García-Marín, David | - |
dc.date | 2022-01-27 | - |
dc.identifier | https://revistas.ucm.es/index.php/DCIN/article/view/77472 | - |
dc.identifier | 10.5209/dcin.77472 | - |
dc.description | Automated fact-checking consists of automatically determining the veracity of a claim by applying existing artificial intelligence technologies to classify it into one of the categories commonly used by human fact-checkers (true, misleading, false, etc.). This paper presents the first systematic literature review in Spanish on the evolution of research on this topic. It also aims to analyze the level of accuracy of algorithmic solutions and the impact of published work, using descriptive and inferential statistical treatments (chi-square and Kruskal-Wallis tests). According to our results, the highest volume of contributions was concentrated in the last three years, mainly from the Asian region and United States. Papers proposing integrated algorithmic methods or systems predominate. Studies on linguistic models, which still have several limitations and below-average effectiveness, are in the majority. There is little attention to models based on image analysis, and the presence of fake audio detection algorithms is practically nonexistent. In line with previous work, our study concludes that there are no statistically significant differences in the level of accuracy of the diverse algorithmic models proposed, despite their different degrees of technical complexity. | en-US |
dc.description | El fact-checking automatizado consiste en la comprobación automática de la veracidad de una información aplicando las tecnologías de inteligencia artificial existentes para clasificarla en alguna de las categorías comúnmente usadas por los fact-checkers humanos (verdadero, engañoso, falso, etc.). Este trabajo presenta el primer análisis bibliométrico en castellano -de tipo cuantitativo- sobre la evolución y los países de procedencia de la investigación sobre esta práctica. Asimismo, pretende analizar el nivel de precisión de las soluciones algorítmicas y el impacto de los trabajos publicados, utilizando para ello tratamientos estadísticos descriptivos e inferenciales (pruebas de chi cuadrado y test de Kruskal-Wallis). De acuerdo con nuestros resultados, en los últimos tres años se concentra el mayor volumen de aportaciones, que proceden mayoritariamente de la región asiática y Estados Unidos. Predominan los trabajos que proponen métodos o sistemas algorítmicos integrados. Son mayoritarios los estudios sobre modelos lingüísticos, que presentan aún varias limitaciones y una efectividad inferior a la media. Se observa una reducida atención hacia los modelos basados en el análisis de imágenes, y resulta prácticamente nula la presencia de algoritmos de detección de audios falsos. En línea con trabajos anteriores, nuestro estudio concluye que no existen diferencias estadísticamente significativas en el nivel de precisión de los diversos modelos algorítmicos propuestos, a pesar de sus diferentes grados de complejidad técnica. | es-ES |
dc.format | application/pdf | - |
dc.language | spa | - |
dc.publisher | Ediciones Complutense | es-ES |
dc.relation | https://revistas.ucm.es/index.php/DCIN/article/view/77472/4564456559646 | - |
dc.relation | /*ref*/Aleixandre-Benavent, R., Castelló-Cogollos, L. y Valderrama-Zurián, J.C. (2020). Información y comunicación durante los primeros meses de Covid-19. Infodemia, desinformación y papel de los profesionales de la información. Profesional de la información, 29(4), e290408. https://doi.org/10.3145/epi.2020.jul.08. | - |
dc.relation | /*ref*/Amjad, M., Sidorov, G., Zhila, A., Gómez-Adorno, H., Voronkov, I. y Gelbukh, A. (2020). “Bend the truth”: Benchmark dataset for fake news detection in Urdu language and its evaluation. Journal of Intelligent & Fuzzy Systems, 39(2), 2457-2469. https://doi.org/10.3233/JIFS-179905. | - |
dc.relation | /*ref*/Antonakaki, D., Fragopoulou, P. y Ioannidis, S. (2021). A survey of Twitter research: Data model, graph structure, sentiment analysis and attacks. Expert Systems with Applications, 164, 114006. https://doi.org/10.1016/j.eswa.2020.114006. | - |
dc.relation | /*ref*/Aparici, R. y García-Marín, D. (2019). La posverdad. Una cartografía de los medios, las redes y la política. Gedisa. | - |
dc.relation | /*ref*/Caldevilla-Domínguez, D. y García-García, E. (2020). Profesionales y posverdad: La responsabilidad colectiva como arma contra la falacia digitalizada. aDResearch, 21(21), 70-83. https://doi.org/10.7263/adresic-021-04. | - |
dc.relation | /*ref*/Choraś, M., Demestichas, K., Giełczyk, A., Herrero, Á., Ksieniewicz, P., Remoundou, K., Urda, D. y Woźniak, M. (2020). Advanced Machine Learning techniques for fake news (online disinformation) detection: A systematic mapping study. Applied Soft Computing, 107050. https://doi.org/10.1016/j.asoc.2020.107050. | - |
dc.relation | /*ref*/Dale, R. (2017). NLP in a post-truth world. Natural Language Engineering, 23(2), 319– 324. https://doi.org/10.1017/S1351324917000018. | - |
dc.relation | /*ref*/Elías, C. (2021). El periodismo como herramienta contra las fake news. En C. Elías y D. Teira (Eds.), Manual de periodismo y verificación de noticias en la era de las fake news (pp. 19-57). Editorial UNED. | - |
dc.relation | /*ref*/García-Marín, D. (2021). Las fake news y los periodistas de la generación z. Soluciones post-millennial contra la desinformación. Vivat Academia. Revista de Comunicación, 154, 37-63. http://doi.org/10.15178/va.2021.154.e1324. | - |
dc.relation | /*ref*/García-Ortega, A. y García-Avilés, J.A. (2021). Uso del diseño lúdico para combatir la desinformación. Revista Icono 14, 19(1), 179-204. https://doi.org/10.7195/ri14.v19i1.1598. | - |
dc.relation | /*ref*/Gereme, F., Zhu, W., Ayall, T. y Alemu, D. (2021). Combating fake news in “low-resource” languages: Amharic fake news detection accompanied by resource crafting. Information, 12(1), 20. https://doi.org/10.3390/info12010020. | - |
dc.relation | /*ref*/Goldani, M. H., Momtazi, S. y Safabakhsh, R. (2021). Detecting fake news with capsule neural networks. Applied Soft Computing, 101, 106991. https://doi.org/10.1016/j.asoc.2020.106991. | - |
dc.relation | /*ref*/Goldani, M. H., Safabakhsh, R. y Momtazi, S. (2021). Convolutional neural network with margin loss for fake news detection. Information Processing & Management, 58(1), 102418. https://doi.org/10.1016/j.ipm.2020.102418. | - |
dc.relation | /*ref*/Horne, B. y Adali, S. (2017). This just in: Fake news packs a lot in title, uses simpler, repetitive content in text body, more similar to satire than real news. Proceedings of the International AAAI Conference on Web and Social Media, 11(1). https://cutt.ly/eQqMXwo. | - |
dc.relation | /*ref*/Huang, Y.F. y Chen, P.H. (2020). Fake news detection using an ensemble learning model based on self-adaptive harmony search algorithms. Expert Systems with Applications, 159, 113584. https://doi.org/10.1016/j.eswa.2020.113584. | - |
dc.relation | /*ref*/Ilias, L. y Roussaki, I. (2021). Detecting malicious activity in Twitter using deep learning techniques. Applied Soft Computing, 107, 107360. https://doi.org/10.1016/j.asoc.2021.107360. | - |
dc.relation | /*ref*/Kasban, H. y Nassar, S. (2020). An efficient approach for forgery detection in digital images using Hilbert–Huang transform. Applied Soft Computing, 97, 106728. https://doi.org/10.1016/j.asoc.2020.106728. | - |
dc.relation | /*ref*/Kaur, S., Kumar, P. y Kumaraguru, P. (2020). Automating fake news detection system using multi-level voting model. Soft Computing, 24(12), 9049-9069. https://doi.org/10.1007/s00500-019-04436-y | - |
dc.relation | /*ref*/Lease, M. (2018). Fact Checking and Information Retrieval. DESIRES, 97-98. https://cutt.ly/rQqMTv3. | - |
dc.relation | /*ref*/López-García, X., Silva-Rodríguez, A., Vizoso-García, A.A. Westlund, O. y Canavilhas, J. (2019). Periodismo móvil: Revisión sistemática de la producción científica. Comunicar, 27, 9-18. https://doi.org/10.3916/C59-2019-01. | - |
dc.relation | /*ref*/Magdy, A. y Wanas, N. (2010). Web-based statistical fact checking of textual documents. Proceedings of the 2nd international workshop on Search and mining user-generated contents, 103-110. https://cutt.ly/FQqBjDl. | - |
dc.relation | /*ref*/Meneses-Silva, C.V., Silva-Fontes, R. y Colaço-Júnior, M. (2021). Intelligent fake news detection: a systematic mapping. Journal of applied security research, 16(2), 168-189. https://doi.org/10.1080/19361610.2020.1761224. | - |
dc.relation | /*ref*/McIntyre, L. (2018). Post-truth. MIT Press. | - |
dc.relation | /*ref*/Mouratidis, D., Nikiforos, M.N. y Kermanidis, K. L. (2021). Deep Learning for Fake News Detection in a Pairwise Textual Input Schema. Computation, 9(2), 20. https://doi.org/10.3390/computation9020020. | - |
dc.relation | /*ref*/Núñez-Mussa, E. (2020). Cómo verificar sin expertos y llegar a las grandes ligas. Obra Digital, 18, 85-101. https://doi.org/10.25029/od.2020.236.18. | - |
dc.relation | /*ref*/Ongsulee, P. (2017). Artificial intelligence, machine learning and deep learning. 2017 15th International Conference on ICT and Knowledge Engineering (ICT&KE), 1-6. https://cutt.ly/VQq1bg0. | - |
dc.relation | /*ref*/Oshikawa, R., Qian, J. y Wang, W.Y. (2018). A survey on natural language processing for fake news detection. arXiv, 1811.00770. https://cutt.ly/qQq1upc. | - |
dc.relation | /*ref*/Polanco, X. (1997). Infometría e Ingeniería del Conocimiento: Exploración de Datos y Análisis de la Información en vista del Descubrimiento de Conocimientos. En H. Jaramillo y M. Albornoz (Compiladores), El universo de la medición: La perspectiva de la Ciencia y la Tecnología (pp. 335-350). COLCIENCIAS, CYTED, RICYT. Tercer Mundo Editores. | - |
dc.relation | /*ref*/Posadas-Durán, J.P., Gómez-Adorno, H., Sidorov, G. y Moreno-Escobar, J. J. (2019). Detection of fake news in a new corpus for the Spanish language. Journal of Intelligent & Fuzzy Systems, 36(5), 4869-4876. https://doi.org/10.3233/JIFS-179034. | - |
dc.relation | /*ref*/Potthast, M., Kiesel, J., Reinartz, K., Bevendorff, J. y Stein, B. (2017). A stylometric inquiry into hyperpartisan and fake news. arXiv,1702.05638. https://cutt.ly/eQqM8Yr. | - |
dc.relation | /*ref*/Puebla-Martínez, B., Del Campo, E. y Pérez-Cuadrado, P. (2018). Análisis bibliométrico de la revista index.comunicación (2011-2017). Estrategias de posicionamiento inicial. En R. Repiso, J. Guallar y J. M. de Pablos (Eds.), Revistas científicas de Ciencias de la Información en el abismo (pp. 39-64). Egregius Ediciones y Universidad de Zaragoza. | - |
dc.relation | /*ref*/Ruiz-Rico, M. (2020). Truth as Literature: Ethics of Journalism and Reality in the Digital Society. Estudios sobre el mensaje periodístico, 26(1), 307-315. https://doi.org/10.5209/esmp.67309. | - |
dc.relation | /*ref*/Sadin, E. (2017). La humanidad aumentada. La administración digital del mundo. Caja Negra Editora. | - |
dc.relation | /*ref*/Sahoo, S.R. y Gupta, B.B. (2021). Multiple features based approach for automatic fake news detection on social networks using deep learning. Applied Soft Computing, 100, 106983. https://doi.org/10.1016/j.asoc.2020.106983. | - |
dc.relation | /*ref*/Saquete, E., Tomás, D., Moreda, P., Martínez-Barco, P. y Palomar, M. (2020). Fighting post-truth using natural language processing: A review and open challenges. Expert systems with applications, 141, 112943. https://doi.org/10.1016/j.eswa.2019.112943. | - |
dc.relation | /*ref*/Schuster, T., Schuster, R., Shah, D. J. y Barzilay, R. (2020). The limitations of stylometry for detecting machine-generated fake news. Computational Linguistics, 46(2), 499-510. https://doi.org/10.1162/COLI a 00380. | - |
dc.relation | /*ref*/Silva, R.M., Santos, R.L., Almeida, T.A. y Pardo, T.A. (2020). Towards automatically filtering fake news in Portuguese. Expert Systems with Applications, 146, 113199. https://doi.org/10.1016/j.eswa.2020.113199. | - |
dc.relation | /*ref*/Thaher, T., Saheb, M., Turabieh, H. y Chantar, H. (2021). Intelligent Detection of False Information in Arabic Tweets Utilizing Hybrid Harris Hawks Based Feature Selection and Machine Learning Models. Symmetry, 13(4). https://doi.org/10.3390/sym13040556. | - |
dc.relation | /*ref*/Zervopoulos, A., Alvanou, A. G., Bezas, K., Papamichail, A., Maragoudakis, M. y Kermanidis, K. (2021). Deep learning for fake news detection on Twitter regarding the 2019 Hong Kong protests. Neural Computing and Applications, 1-14. https://doi.org/10.1007/s00521-021-06230-0. | - |
dc.rights | Derechos de autor 2021 Documentación de las Ciencias de la Información | es-ES |
dc.source | Documentación de las Ciencias de la Información; Vol. 45 No. 1 (2022): Periodismo y algoritmos: de la era de la información a la era del algoritmo; 7-16 | en-US |
dc.source | Documentación de las Ciencias de la Información; Vol. 45 Núm. 1 (2022): Periodismo y algoritmos: de la era de la información a la era del algoritmo; 7-16 | es-ES |
dc.source | 1988-2890 | - |
dc.source | 0210-4210 | - |
dc.subject | automated fact-checking | en-US |
dc.subject | disinformation | en-US |
dc.subject | fake news | en-US |
dc.subject | systematic literature review | en-US |
dc.subject | algorithms | en-US |
dc.subject | fact-checking automatizado | es-ES |
dc.subject | desinformación | es-ES |
dc.subject | fake news | es-ES |
dc.subject | revisión sistemática | es-ES |
dc.subject | algoritmos | es-ES |
dc.title | Algorithmic models and automated fact-checking. A systematic literature review | en-US |
dc.title | Modelos algorítmicos y fact-checking automatizado. Revisión sistemática de la literatura | es-ES |
dc.type | info:eu-repo/semantics/article | - |
dc.type | info:eu-repo/semantics/publishedVersion | - |
Aparece en las colecciones: | Servicio Documentación Multimedia. Sección Departamental de Biblioteconomía y Documentación. Universidad Complutense de Madrid - SDM - Cosecha |
Ficheros en este ítem:
No hay ficheros asociados a este ítem.
Los ítems de DSpace están protegidos por copyright, con todos los derechos reservados, a menos que se indique lo contrario.