Red de Bibliotecas Virtuales de Ciencias Sociales en
América Latina y el Caribe
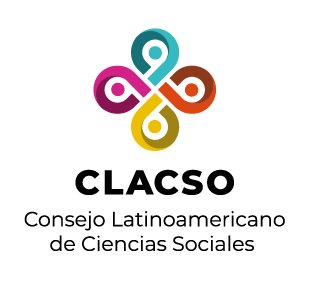
Por favor, use este identificador para citar o enlazar este ítem:
https://biblioteca-repositorio.clacso.edu.ar/handle/CLACSO/109725
Registro completo de metadatos
Campo DC | Valor | Lengua/Idioma |
---|---|---|
dc.creator | Rodríguez Cano, Norman Simón | - |
dc.date | 2018-07-01 | - |
dc.date.accessioned | 2022-03-23T15:10:28Z | - |
dc.date.available | 2022-03-23T15:10:28Z | - |
dc.identifier | https://revistas.unal.edu.co/index.php/ede/article/view/75382 | - |
dc.identifier | 10.15446/ede.v28n53.75382 | - |
dc.identifier.uri | http://biblioteca-repositorio.clacso.edu.ar/handle/CLACSO/109725 | - |
dc.description | Policy evaluation is a discipline dedicated to the qualitative and quantitative examination of the decisions made by governments to provide solutions for pressing social issues. Its methods and concepts come from a variety of fields, such as economics, political science, statistics and computer science, among others. This paper provides the historical and methodological background of the current trends in policy evaluation, focusing on formative evaluation and impact evaluation. It also reflects on the potential applications of artificial intelligence and big data in this discipline | en-US |
dc.description | La evaluación de políticas públicas es una disciplina que tiene como objeto el examen cualitativo y cuantitativo de las decisiones tomadas por los gobiernos para resolver problemáticas sociales. Metodológica y conceptualmente, se nutre de la economía, la ciencia política, la estadística y la computación, entre otras ciencias. En este artículo se contextualizan histórica y metodológicamentelas tendencias actuales en la evaluación de políticas públicas, especialmente en la evaluación de diseño y la evaluación de impacto. También se reflexiona acerca de las potencialidades de la inteligencia artificial y el big data para esta disciplina. | es-ES |
dc.format | application/pdf | - |
dc.format | application/xml | - |
dc.language | spa | - |
dc.publisher | Universidad Nacional de Colombia - Sede Medellín - Facultad de Ciencias Humanas y Económicas - Departamento de Economía | es-ES |
dc.relation | https://revistas.unal.edu.co/index.php/ede/article/view/75382/69781 | - |
dc.relation | https://revistas.unal.edu.co/index.php/ede/article/view/75382/70088 | - |
dc.relation | /*ref*/Basu, K. (2013). The Method of Randomization and the Role of Reasoned Intuition.The World Bank Group. Recuperado de https://papers.ssrn.com/sol3/papers.cfm?abstract_id=2367082 | - |
dc.relation | /*ref*/Bates, M. y Glennerster, R. (2017). The Generalizability Puzzle. Stanford Social Innovation Review, 15(3). https://ssir.org/articles/entry/the_generalizability_puzzle | - |
dc.relation | /*ref*/Berry, D. (2006). Bayesian Clinical Trials. Nature Reviews. Drug Discovery, 5, 27-36. https://www.stat.ubc.ca/~rollin/teach/bar/Berry2006NatureReviewsDD.pdf | - |
dc.relation | /*ref*/Blumenstock, J., Cadamuro, G. y On, R. (2015). Predicting Poverty and Wealth from Mobile Phone Metadata. Science, 350(6264), 1073-1076. http://sampleu.ec.unipi.it/wp-content/uploads/2015/12/Science-2015-Blumenstock-1073-6.pdf | - |
dc.relation | /*ref*/Brodersen, K. (23 de septiembre de 2015). Causal Attribution in an Era of Big Time-Series Data. [Mensaje en un blog]. Recuperado de http://www.unofficialgoogledatascience.com/2015/09/causal-attribution-in-era-of-big-time.html | - |
dc.relation | /*ref*/Brodersen, K., Galluser, F., Koehler, J., Remy, N. y Scott, S. (2015). Inferring Causal Impact Using Bayesian Structural Time-Series Models. The Annals of Applied Statistics, 9(1), 247-274. https://arxiv.org/pdf/1506.00356.pdf | - |
dc.relation | /*ref*/Brodersen, K. y Hauser, A. (2017). An R Package for Causal Inference Using Bayesian Structural Time-Series Models. CRAN. Recuperado de https://cran.r-project.org/web/packages/CausalImpact/vignettes/CausalImpact.html | - |
dc.relation | /*ref*/Cartwright, N. (2014). Evidence: For Policy And Wheresoever Rigor is a Must. Londres: The London School of Economics and Political Science. | - |
dc.relation | /*ref*/Cartwright, N. y Munro, E. (2010). The Limitations of Randomized Controlled Trials in Predicting Effectiveness. Journal of Evaluation in Clinical Practice, 16(2), 260-266. https://doi.org/10.1111/j.1365-2753.2010.01382.x | - |
dc.relation | /*ref*/Cencini, A. (2005). Macroeconomic Foundations of Macroeconomics. Nueva York: Routledge. | - |
dc.relation | /*ref*/Donaldson, D. y Storeygard, A. (2016). The View From Above: Applications of Satellite Data in Economics. Journal of Economic Perspectives, 30(4), 171-198. http://dave-donaldson.com/wpcontent/uploads/2016/10/Donaldson_Storeygard_JEP.pdf | - |
dc.relation | /*ref*/Duflo, E. y Banerjee, A. (2012). Repensar la pobreza: un giro radical en la lucha contra la desigualdad global. Madrid: Taurus. | - |
dc.relation | /*ref*/Duflo, E. y Banerjee, A. (eds.). (2017). Handbook of Field Experiments, Volume 1. Amsterdan: Elsevier. | - |
dc.relation | /*ref*/Fienberg, S. (2011). Subjective Bayesian Models and Methods in Public Policy and Government Settings. Statistical Science, 26(2), 212-226. https://doi.org/10.1214/10-STS331 | - |
dc.relation | /*ref*/Fisher, R. (1937). The Design of Experiments. Edimburgo: Oliver and Boyd. | - |
dc.relation | /*ref*/Forrester, J. (1969). Urban Dynamics. Cambridge: M.I.T. Press. | - |
dc.relation | /*ref*/Forrester, J. (1971). World Dynamics. Cambridge: M.I.T. Press. | - |
dc.relation | /*ref*/Friedmann, J. (1987). Planning in the Public Domain: From Knowledge to Action. Princeton: Princeton University Press. | - |
dc.relation | /*ref*/Fukuyama, F. (1992). The End of History and The last Man. Nueva York : The Free Press. Ghaffarzadegan, N., Lyneis, J. y Richardson, G. (2011). How Small System Dynamics Models Can Help the Public Policy Process. System Dynamics Review, 27(1), 22-44. https://doi.org/10.1002/sdr.442 | - |
dc.relation | /*ref*/Giabbanelli, P. y Crutzen, R. (2017). Using Agent-Based Models to Develop Public Policy about Food Behaviours: Future Directions and Recommendations. Computational and Mathematical Methods in Medicine, 2017, 1-12. https://doi.org/10.1155/2017/5742629 | - |
dc.relation | /*ref*/Ge, F., Wei, Z., Lu, Y., Tian, Y. y Li, L. (2012). Decentralized Coordination Of Autonomous Swarms Inspired by Chaotic Behavior of Ants. Nonlinear Dynamics, 70(1), 571-584. https://doi.org/10.1007/s11071-012-0478-z | - |
dc.relation | /*ref*/Gentile, J., Glazner, C. y Koehler, M. (2015). Simulation Models for Public Policy. En B. Furtado, P. Sakowski y M. Tóvolli (Eds.), Modeling Complex Systems For Public Policies (pp.73-83). Brasilia: Instituto de Pesquisa Econômica Aplicada – IPEA. | - |
dc.relation | /*ref*/Gertler, P., Martínez, S., Premand, P., Rawlings, L. y Vermeersch, C. (2017). La evaluación de impacto en la práctica: segunda edición. Washington: Banco Mundial – Banco Interamericano de Desarrollo. | - |
dc.relation | /*ref*/Glaeser, E., Duke, S., Luca, M. y Naik, N. (2015). Big Data and Big Cities: The Promises and Limitations of Improved Measures of Urban Life. National Bureau of Economic Research. Recuperado de http://www.nber.org/papers/w21778 | - |
dc.relation | /*ref*/Glennerster, R. (2017). When Do Innovation and Evidence Change Lives? [Mensaje en un blog]. Recuperado de http://runningres.com/blog/2017/11/9/when-do-innovation-and-evidence-change-lives | - |
dc.relation | /*ref*/Haggenson, N. (1990). System Dynamics Combined with Monte Carlo Simulation. Proceeds of the 1990 System Dynamics conference. Recuperado de https://www.systemdynamics.org/assets/conferences/1990/proceed/pdfs/hagen468.pdf | - |
dc.relation | /*ref*/Helbing, D. (Ed.). (2012). Agent-Based Modeling. Social Self-Organization. Understanding Complex Systems. Berlín: Springer. | - |
dc.relation | /*ref*/Iacus, S., King, G. y Porro, G. (2012). Causal Inference without Balance Checking: Coarsened Exact Matching. Political Analysis, 20(1), 1-24. https://doi.org/10.1093/pan/mpr013 | - |
dc.relation | /*ref*/Jean, N., Burke, M., Xie, M., Davis, M., Lobell, D. y Ermon, S. (2016). Combining Satellite Imagery and Machine Learning to Predict Poverty. Science, 353(6301), 790-794. https://doi.org/10.1126/science.aaf7894 | - |
dc.relation | /*ref*/Keane, M. y Wolpin, K. (1994). The Solution and Estimation of Discrete Choice Dynamic Programming Models by Simulation: Monte Carlo Evidence. Review of Economics and Statistics, 74(4), 648-672. | - |
dc.relation | /*ref*/Kennedy, W. G., Ihara, E. S., Tompkins, C. J., Inoue, M. y Wolf-Branigin, M. E. (2015). Computational Modeling of Caregiver Stress. Policy and Complex Systems, 2(1), 31-44. https://doi.org/10.18278/jpcs.2.1.5 | - |
dc.relation | /*ref*/King, G. y Nielsen, R. (2016). Why Propensity Scores Should Not Be Used for Matching. Gary King. Recuperado de https://gking.harvard.edu/files/gking/files/psnot.pdf | - |
dc.relation | /*ref*/Lee, D. (2005). An Estimable Dynamic General Equilibrium Model of Work, Schooling, and Occupational Choice. International Economic Review, 46(1), 1-34. https://www.jstor.org/stable/3663586?seq=1#page_scan_tab_contents | - |
dc.relation | /*ref*/Lee, J. y Chu, C. (2012). Bayesian Clinical Trials in Action. Statistics in Medicine, 31(25), 2955-2972. https://doi.org/10.1002/sim.5404 | - |
dc.relation | /*ref*/Meadows, D. H., Meadows, D. L., Randers, J. y Behrens, W. (1972). The Limits to Growth. Nueva York: Universe Books. | - |
dc.relation | /*ref*/Michaloupoulos, C. (2012). A Bayesian Reanalysis of Results from the Hard-to-Employ Demonstration and Evaluation Project. Office of Planning, Research and Evaluation (OPRE) – Administration for Children and Families – U.S. Department of Health and Human Services – Office of the Assistant Secretary for Planning and Evaluation – U.S. Department of Health and Human Services https://www.acf.hhs.gov/sites/default/files/opre/bayesian_reanalysis.pdf | - |
dc.relation | /*ref*/Mullainathan, S. (2 de abril de 2016). Satellite Images Can Pinpoint Poverty Where Surveys Can’t. The New York Times. Recuperado de https://www.nytimes.com/2016/04/03/upshot/satellite-images-can-pinpoint-poverty-where-surveys-cant.html | - |
dc.relation | /*ref*/Muralidharan, O., Cardin, N., Phillips, T. y Najmi, A. (31 de enero de 2017). Causality in Machine Learning. [Mensaje en un blog]. Recuperado de http://www.unofficialgoogledatascience.com/2017/01/causality-in-machine-learning.html | - |
dc.relation | /*ref*/Orloff, J. y Bloom, J. (2014). Comparison of Frequentist and Bayesian Inference. Massachusetts Institute of Technology. Recuperado de https://ocw.mit.edu/courses/mathematics/18-05-introduction-to-probability-and-statistics-spring-2014/readings/MIT18_05S14_Reading20.pdf | - |
dc.relation | /*ref*/Pearl, J. (2001). Causal Inference in Statistics: A Gentle Introduction. Submitted to ComputingScience and Statistics, Proceedings of Interface, 33(1), 1-20. http://ftp.cs.ucla.edu/pub/stat_ser/R289.pdf | - |
dc.relation | /*ref*/Pearl, J. (2003). Causality: Models, Reasoning and Inference. Econometric Theory, 19(4), 675-685. Pritchett, L. y Sandfur, J. (2013). Context Matters for Size: Why External Validity Claims and Development Practice Don’t Mix. Center for Global Development. Recuperado de https://www.cgdev.org/sites/default/files/context-matters-for-size_1.pdf | - |
dc.relation | /*ref*/Ramsey, F. (1928). A Mathematical Theory of Saving. The Economic Journal, 38(152), 543-559. | - |
dc.relation | /*ref*/Rand, W. (2017). Introduction to Agent-Based Modeling. Complexity Explorer – Santa Fe Institute. Recuperado de https://abm.complexityexplorer.org | - |
dc.relation | /*ref*/Ros, J. (2012). La Teoría General de Keynes y la macroeconomía moderna. Investigación económica, 71(279), 19-37. | - |
dc.relation | /*ref*/Salazar, C. (2009). La evaluación y el análisis de políticas públicas. Ópera, 9, 23-51. | - |
dc.relation | /*ref*/Schelling, T. (1978). Micromotives and Macrobehavior. Nueva York: W.W Norton. | - |
dc.relation | /*ref*/Simon, R. (1999). Bayesian Design and Analysis of Active Control Clinical Trials. Biometrics, 55(2), 484-487. | - |
dc.relation | /*ref*/Spiegelhalter, D. y Freedman, L. (1994). Bayesian Approaches to Randomized Trials. Journal of the Royal Statistical Society. Series A (Statistics in Society), 157(3), 357-416. | - |
dc.relation | /*ref*/Tang, D., Agarwal, A., O’Brien, D. y Meyer, M. (2010). Overlapping Experiment Infrastructure: More, Better, Faster Experimentation. Google. Recuperado de https://research.google.com/pubs/pub36500.html | - |
dc.relation | /*ref*/Todd, P. y Wolpin, K. (2008). Ex-ante Evaluation of Social Programs. Annales d’Économie et de Statistique, 91/92, 263-291. | - |
dc.relation | /*ref*/Wilensky, U. y Rand, W. (2015). An Introduction to Agent-Based Modeling: Modeling Natural, Social, and Engineered Complex Systems with NetLogo. Cambridge: The MIT Press. | - |
dc.rights | Derechos de autor 2018 Ensayos de Economía | es-ES |
dc.rights | https://creativecommons.org/licenses/by-nc-nd/4.0 | es-ES |
dc.source | Ensayos de Economía; Vol. 28 No. 53 (2018); 15-35 | en-US |
dc.source | Ensayos de Economía; Vol. 28 Núm. 53 (2018); 15-35 | es-ES |
dc.source | Ensayos de Economía; Vol. 28 No. 53 (2018); 15-35 | fr-CA |
dc.source | 2619-6573 | - |
dc.source | 0121-117X | - |
dc.subject | Public policy | en-US |
dc.subject | evaluation | en-US |
dc.subject | development | en-US |
dc.subject | bayesian networks | en-US |
dc.subject | computer-based modelling | en-US |
dc.subject | randomised experiment | en-US |
dc.subject | Economics | en-US |
dc.subject | Development economics | en-US |
dc.subject | Policy evaluation | en-US |
dc.subject | políticas públicas | es-ES |
dc.subject | evaluación | es-ES |
dc.subject | desarrollo | es-ES |
dc.subject | redes bayesianas | es-ES |
dc.subject | modelación computarizada | es-ES |
dc.subject | experimento aleatorizado | es-ES |
dc.subject | Economía | es-ES |
dc.subject | Economía del desarrollo | es-ES |
dc.subject | Evaluación de políticas públicas | es-ES |
dc.subject | Économie | fr-CA |
dc.subject | Économie du développement | fr-CA |
dc.subject | Évaluation des politiques publiques | fr-CA |
dc.title | Current Trends in Public Policy Evaluation | en-US |
dc.title | Tendencias actuales en la evaluación de políticas públicas | es-ES |
dc.type | info:eu-repo/semantics/article | - |
dc.type | info:eu-repo/semantics/publishedVersion | - |
Aparece en las colecciones: | Facultad de Ciencias Humanas y Económicas - FCHE/UNAL - Cosecha |
Ficheros en este ítem:
No hay ficheros asociados a este ítem.
Los ítems de DSpace están protegidos por copyright, con todos los derechos reservados, a menos que se indique lo contrario.