Red de Bibliotecas Virtuales de Ciencias Sociales en
América Latina y el Caribe
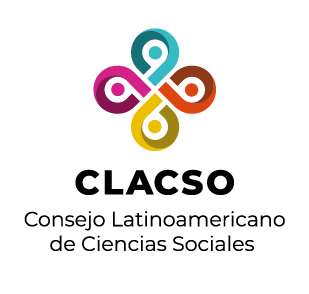
Por favor, use este identificador para citar o enlazar este ítem:
https://biblioteca-repositorio.clacso.edu.ar/handle/CLACSO/246804
Título : | Ecophysiology modeling by artificial neural networks for different spacings in eucalypt Ecophysiology modeling by artificial neural networks for different spacings in eucalypt |
Palabras clave : | biomassa de lenho;densidade de plantio;ecofisiologia;prognose;RNA |
Editorial : | Federal University of Piauí |
Descripción : | Growth and production models are widely used to predict yields and support forestry decisions. Artificial Neural Networks (ANN) are computational models that simulate the brain and nervous system human functions, with a memory capable of establishing mathematical relationships between independent variables to estimate the dependent variables. This work aimed to evaluate the efficiency of eucalypt biomass modeling under different spacings using Multilayer Perceptron networks, trained through the backpropagation algorithm. The experiment was installed in randomized block, and the effect of five planting spacings was studied in three blocks: T1 – 3.0 x 0.5 m; T2 – 3.0 x 1.0 m; T3 – 3.0 x 1.5 m; T4 – 3.0 x 2.0 m e T5 – 3.0 x 3.0 m. A continuous forest inventory was carried out at the ages of 48, 61, 73, 85 and 101 months. The leaf area, leaf perimeter and specific leaf area were measured at 101 months in one sample tree per experimental unit. Two thousand ANN were trained, using all inventoried trees, to estimate the eco-physiological attributes and the prognosis of the wood biomass. The artificial neural networks modeling was adequate to estimate eucalypt wood biomass, according to age and under different spacings, using the diameter-at-breast-height and leaf perimeter as predictor variables. Growth and production models are widely used to predict yields and support forestry decisions. Artificial Neural Networks (ANN) are computational models that simulate the brain and nervous system human functions, with a memory capable of establishing mathematical relationships between independent variables to estimate the dependent variables. This work aimed to evaluate the efficiency of eucalypt biomass modeling under different spacings using Multilayer Perceptron networks, trained through the backpropagation algorithm. The experiment was installed in randomized block, and the effect of five planting spacings was studied in three blocks: T1 – 3.0 x 0.5 m; T2 – 3.0 x 1.0 m; T3 – 3.0 x 1.5 m; T4 – 3.0 x 2.0 m e T5 – 3.0 x 3.0 m. A continuous forest inventory was carried out at the ages of 48, 61, 73, 85 and 101 months. The leaf area, leaf perimeter and specific leaf area were measured at 101 months in one sample tree per experimental unit. Two thousand ANN were trained, using all inventoried trees, to estimate the eco-physiological attributes and the prognosis of the wood biomass. The artificial neural networks modeling was adequate to estimate eucalypt wood biomass, according to age and under different spacings, using the diameter-at-breast-height and leaf perimeter as predictor variables. |
URI : | https://biblioteca-repositorio.clacso.edu.ar/handle/CLACSO/246804 |
Otros identificadores : | https://comunicatascientiae.com.br/comunicata/article/view/2741 10.14295/cs.v9i3.2741 |
Aparece en las colecciones: | Núcleo de Pesquisa sobre Crianças, Adolescestes e Jovens - Universidade Federal do Piauí - NUPEC/UFPI - Cosecha |
Ficheros en este ítem:
No hay ficheros asociados a este ítem.
Los ítems de DSpace están protegidos por copyright, con todos los derechos reservados, a menos que se indique lo contrario.